Join us
JOIN OUR COMMUNITY OF DATA EXPERTS
Predicting and shaping the futureWhere others see numbers, I see a glimpse of the world of tomorrow
Lucile wasn’t necessarily looking for an experience working in a bank. “It wasn’t a sector that appealed to me,” she tells us. And yet, her internship turned into a short-term, and then permanent contract. For her, it’s the variety of missions and international opportunities that made all the difference.
Giving meaning to data
It’s a treasure that’s both priceless and unexpected. The data provided by our tools and our users have the power to shape the future. At the Data Lab, it’s up to Lucile and her colleagues to give meaning to the data, to polish this rough diamond and give it its brilliance and clarity.
As a Data Scientist, Lucile uncovers the data’s strategic value. After running a diagnostic on a given subject (an evaluation of ecological policies, for example), she not only puts the data to work for the solutions to be implemented within the group, but also sees the project through to delivery. Data Science concerns every aspect of the bank of tomorrow. That’s why the Data Lab is in constant contact with the business.
This cross-organizational role satisfies both Lucile’s curiosity and her ability to continuously explore new subjects, as well as her rigor in response to the scope of the issues she encounters.
A sector as innovative as this means day-to-day learning.
Data is a sector that moves fast – very fast. And to get ahead of the issues before they even arise, Lucile stays in perpetual learning mode. Note to the self-taught: here, we discover new things on a daily basis.
But beyond self-paced training, the Group is also there to support Lucile and helps her develop her skills and stay on top of the latest innovations.
Mastering new technologies is also part of our day-to-day. “We just finished a project on machine learning,” Caroline, a Business Analyst, says proudly. Being a part of the Data team means always feeling like you’re ahead of your time, if not setting its pace.
330
Data/ IA use casesin 2022
Caroline, Business Analyst and Project Manager
A team that mirrors the sector: young and ambitious
Data Science is in line with the times – and so are the jobs it creates! The Group’s Data teams are a reflection of this fresh trend. Lucile stresses the atmosphere in her young and proactive team whose members support one another in the constant learning that the sector requires.
“We can’t rest on our laurels; it’s non-stop research, supported by the team.”
What a Data Lab team’s day-to-day look like:
- Daily stand-upinspired by agile practices, involving a debrief on the day’s schedule done while standing so it doesn’t drag on!
- Workshopson an as-need basis with developers working on projects.
- Retrospection :feedback following a weeks-long sprint, with a list of areas for improvement.
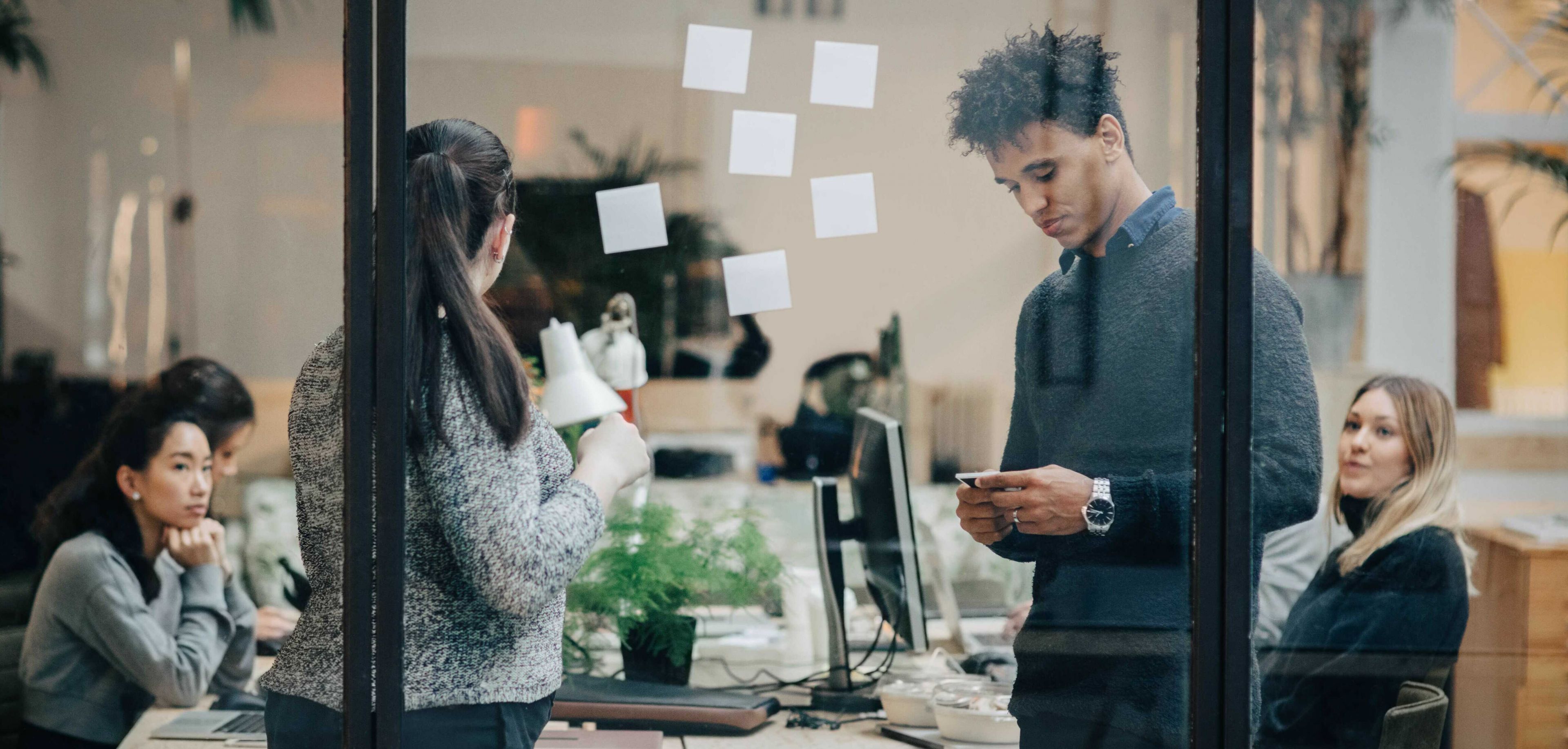
Taking on just causes:
In her new missions, Lucile has associated convictions and vocation: she’s had to build a new Data Lab that places social and ecological responsibilities at the heart of its challenges. The result: new opportunities for activism while shaping the bank of tomorrow.
Caroline loves “spreading her wings cross-organizationally” and working on multiple aspects of life at the Société Générale Group. She regularly takes part in events organized by the Group’s Women in Data Science collective, which seeks to inspire and support women in this domain.
Thriving at the office and at home
Working in a dynamic sector that’s booming doesn’t mean all things professional replace your personal life!
“We’ve all learned to set boundaries,” says Lucile. “My manager told me, ‘I have a life outside of work, and I don’t see why that should be any different for you’”.
Though it’s sometimes hard to let go of an exciting subject, the entire team is there to show the example.
CLOSING REMARKS,BY LUCILE
“I realized that there were tons of subjects within the bank; that allows you to change things up and go from one issue to another. Internal mobility is encouraged, which makes you want to stay for the long haul. I’m about to start a new, completely different job, but I’m staying in the same place. I’m keeping all the benefits and my former colleagues who I can call if I encounter a problem.”